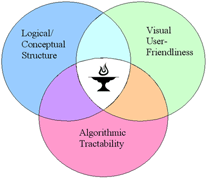 |
When trying to visualize the proximity structure of a high dimensional
pattern, one frequently has to choose between clustering into a hierarchical
tree, or projecting the pattern in two or three dimensions. Conventional
wisdom considers that multi-dimensional scaling (projection) is good
at representing the big picture, whereas hierarchical clustering handles
local details more faithfully. Ideally, one would want a low-dimensional
configuration rendering both the global and local properties; such
that, for example, if one drew between the final points a hierarchical
tree obtained from the original data, this tree would appear simple
and related branches would stay next to each other. Our research adapts
existing scaling algorithms to create such tree-friendly configurations.
More |
|